Transforming Energy Sector Supply Chains: A Deep Dive with Paula Gonzalez on Machine Learning and Digital Innovation
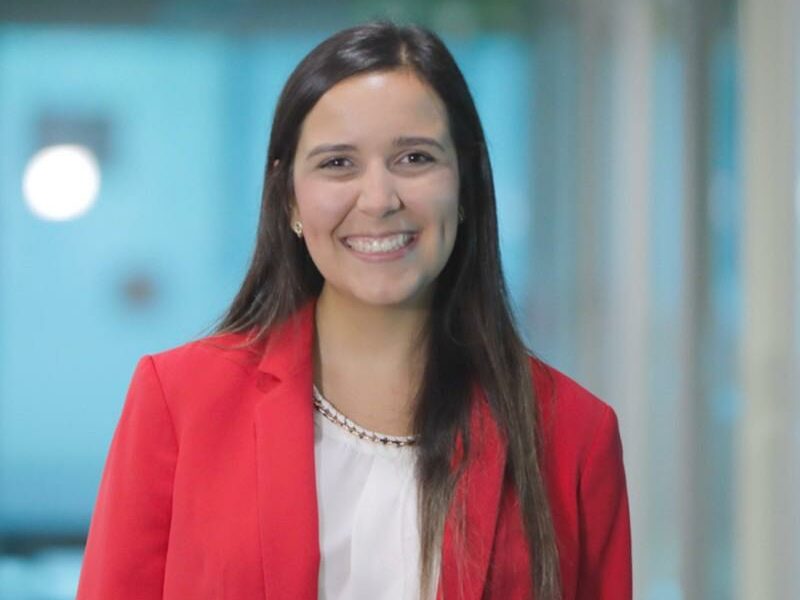
The intersection of machine learning and supply chain management is fundamentally reshaping how energy companies approach procurement, logistics, and operational efficiency. In this in-depth interview, we speak with Paula Gonzalez, a supply chain expert in the energy industry, who brings unique insights from managing complex procurement processes worth over $60 million across multiple operations.
Currently pursuing her MBA at Rice University’s Jones Graduate School of Business, Paula has been at the forefront of digital transformation initiatives, implementing enterprise-wide procurement platforms and developing innovative analytics solutions. Her experience spans upstream, midstream, and downstream operations, where she has leveraged machine learning and predictive analytics to achieve significant cost reductions and operational improvements.
Drawing from her background in production engineering and her MIT certification in Supply Chain Design, Dynamics, Analytics and Technology, Paula offers practical insights on balancing technological innovation with human expertise. In our wide-ranging conversation, she explores how machine learning is revolutionizing supply chain processes, shares strategies for successful digital adoption, and provides a forward-looking perspective on the future of supply chain optimization.
Paula Gonzalez
Paula, you’ve managed procurement processes worth over $60 million across multiple contracts. How has machine learning transformed the way you approach data-driven decision making in procurement?
The way I approach data-driven decision-making in procurement processes has been transformed by enabling predictive analysis, optimizing costs, and automating numerous routine processes through machine learning. Machine learning models provide supply chain practitioners with more accurate forecasts and identify cost-savings opportunities by analyzing historical and real time data. These capabilities of ML improve procurement performance and the strategic decision process by providing more informed and efficient information.
Can you share specific examples of how predictive analytics has revolutionized inventory management and maintenance scheduling in industrial operations? I understand you’ve achieved up to 20% cost reductions through data-driven negotiations.
Predictive analytics has revolutionized industrial operations since it provides more accurate demand forecasting which can be translated into cost-reduction opportunities. For example, an organization that uses ML to analyze historical demand patterns and market trends can predict future demands which translate in optimizing inventory levels and reducing stockouts and overstock situations, increasing efficiency in the process.
Another example could be focused on maintenance scheduling in industrial operations. Predictive analytics leverages data from sensors to anticipate equipment failures in real time. By anticipating potential equipment failures, organizations can prepare for preventive maintenance at optimal times, minimizing costs and minimizing corrective maintenance.
These show how to achieve cost reductions through data-driven in an organization.
In developing analytics dashboards for regional operations, you focus on data accessibility, real-time updates, and customizable views. How do these principles translate into practical advantages for supply chain operations?
Focusing on data accessibility, real-time updates, and customizable views in developing dashboards translates into practical advantages for supply chain operations. Working in supply chain means having multiple stakeholders involved including planning, finance, operations departments, management and suppliers. Accessible data ensures that all stakeholders can easily retrieve and analyze information, reducing bottlenecks and optimizing cycle processes by providing real-time updates that allow practitioners for timely adjustments to address any issues (delivery date delays, stock shortages, etc.). Customizable views enable users and stakeholders to tailor the dashboard to their specific needs, highlighting the most relevant metrics and trends for better monitoring and managing supply chain activities. These principles improve responsiveness, transparency, and strategic planning within supply chain operations.
When implementing enterprise-wide digital solutions, you’ve noted that cultural shift can be more challenging than technical integration. What strategies have proven most effective in driving digital adoption?
Driving digital adoption in an enterprise setting requires addressing the cultural shift just as much as the technical integration. The most effective strategies include comprehensive training programs for a better understanding of the new technologies, empowering workforce with the skills they need, and showing them how the new digital adoption can make their routine jobs easier and more efficient. Clear communication about the benefits and goals of digital transformation helps align everyone with the vision. On the other hand, leadership involvement is crucial; when leaders actively participate and promote digital initiatives, it creates a culture of support and enthusiasm. Additionally, creating a feedback culture where employees can voice concerns and share suggestions ensures continuous improvement and an agile approach. These strategies make the transition smoother and more effective.
You’ve emphasized the importance of balancing advanced technology with human expertise in strategic decision-making. How do you maintain this balance when implementing ML solutions in supply chain operations?
Maintaining the balance between advanced technology and human expertise in supply chain operations is important and it requires three strategies.
First, I always ensure that ML solutions complement rather than replace human decision-making. ML handles data-intensive tasks and provides actionable insights, allowing supply chain practitioners to focus on strategic and complex decisions that require intuition and experience based on data processed by ML.
Second, continuous training keeps the team updated on the latest technologies and enables them to interpret and leverage ML effectively.
Finally, I promote a collaborative environment between technology specialists and supply chain practitioners to work together to align ML solutions with practical and real requirements.
By integrating these approaches, ML enhances human expertise in making informed, strategic decisions.
From your experience reducing manual data input through automated reporting systems, what key metrics should organizations track to demonstrate early wins in their digital transformation journey?
To demonstrate early wins in an organization’s digital transformation journey through automated reporting systems is critical to track key metrics such as data accuracy, processing time, cost savings, productivity, user adoption rate, employee satisfaction, and error rates. Monitoring these metrics provides clear evidence of the benefits gained from automation, such as enhanced data quality, reduced manual labor, and improved efficiency. This quantifiable success can help build momentum and support for further digital initiatives, proving the tangible impact of the transformation on the organization’s overall performance and strategic goals.
For organizations just starting their ML integration journey, you recommend beginning with current data capabilities assessment. What specific areas should they evaluate first?
For organizations just starting their ML integration journey, assessing current data capabilities is essential to build a strong foundation. This assessment should focus on four key areas:
- Evaluate the quality and completeness of their existing data since accurate data is crucial for effective ML models.
- Examine their data infrastructure and data processing capabilities to determine if they can handle the demands of ML tasks.
- Organizations should review their data integration processes to ensure seamless data flow from various sources.
- Organizations should evaluate their team’s ML expertise to identify any training that will support successful implementation.
Organizations can start building a foundation for their ML journey by thoroughly assessing these areas.
Looking ahead, how do you see automated contract management systems and logistics algorithms evolving? What new ML applications do you believe will have the biggest impact on supply chain optimization?
Automated contract management systems and logistics algorithms are set to become increasingly sophisticated. Continuous advancements and developments in ML will enhance capabilities of supply chains, such as real-time risk analysis, automated contract drafting, and predictive analytics for disruptions. One of the most impactful applications in supply chain will be demand forecasting, allowing companies to optimize inventory levels and reduce stockouts. Additionally, using ML for advanced route optimization algorithms will ensure more efficient and cost-effective logistics operations. These innovations will improve decision-making and will transform supply chains into more agile processes.